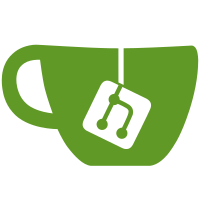
Added a line that in case of not running the peak finder prior to the fit creates empty list of peak positions, ergo it behaves like no peak scenario
231 lines
9.7 KiB
Python
231 lines
9.7 KiB
Python
import numpy as np
|
|
import uncertainties as u
|
|
from lmfit import Model, Parameters
|
|
from scipy.integrate import simps
|
|
|
|
|
|
def bin_data(array, binsize):
|
|
if isinstance(binsize, int) and 0 < binsize < len(array):
|
|
return [
|
|
np.mean(array[binsize * i : binsize * i + binsize])
|
|
for i in range(int(np.ceil(len(array) / binsize)))
|
|
]
|
|
else:
|
|
print("Binsize need to be positive integer smaller than lenght of array")
|
|
return array
|
|
|
|
|
|
def find_nearest(array, value):
|
|
# find nearest value and return index
|
|
array = np.asarray(array)
|
|
idx = (np.abs(array - value)).argmin()
|
|
return idx
|
|
|
|
|
|
def create_uncertanities(y, y_err):
|
|
# create array with uncertanities for error propagation
|
|
combined = np.array([])
|
|
for i in range(len(y)):
|
|
part = u.ufloat(y[i], y_err[i])
|
|
combined = np.append(combined, part)
|
|
return combined
|
|
|
|
|
|
def fitccl(
|
|
scan,
|
|
guess,
|
|
vary,
|
|
constraints_min,
|
|
constraints_max,
|
|
numfit_min=None,
|
|
numfit_max=None,
|
|
binning=None,
|
|
):
|
|
"""Made for fitting of ccl date where 1 peak is expected. Allows for combination of gaussian and linear model combination
|
|
:param scan: scan in the data dict (i.e. M123)
|
|
:param guess: initial guess for the fitting, if none, some values are added automatically in order (see below)
|
|
:param vary: True if parameter can vary during fitting, False if it to be fixed
|
|
:param numfit_min: minimal value on x axis for numerical integration - if none is centre of gaussian minus 3 sigma
|
|
:param numfit_max: maximal value on x axis for numerical integration - if none is centre of gaussian plus 3 sigma
|
|
:param constraints_min: min constranits value for fit
|
|
:param constraints_max: max constranits value for fit
|
|
:param binning : binning of the data
|
|
:return data dict with additional values
|
|
order for guess, vary, constraints_min, constraints_max:
|
|
[Gaussian centre, Gaussian sigma, Gaussian amplitude, background slope, background intercept]
|
|
examples:
|
|
guess = [None, None, 100, 0, None]
|
|
vary = [True, True, True, True, True]
|
|
constraints_min = [23, None, 50, 0, 0]
|
|
constraints_min = [80, None, 1000, 0, 100]
|
|
"""
|
|
if "peak_indexes" not in scan:
|
|
scan["peak_indexes"] = []
|
|
if len(scan["peak_indexes"]) > 1:
|
|
# return in case of more than 1 peaks
|
|
print("More than 1 peak, scan skipped")
|
|
return
|
|
if binning is None or binning == 0 or binning == 1:
|
|
x = list(scan["om"])
|
|
y = list(scan["Counts"])
|
|
y_err = list(np.sqrt(y)) if scan.get("sigma", None) is None else list(scan["sigma"])
|
|
print(scan["peak_indexes"])
|
|
if not scan["peak_indexes"]:
|
|
centre = np.mean(x)
|
|
else:
|
|
centre = x[int(scan["peak_indexes"])]
|
|
else:
|
|
x = list(scan["om"])
|
|
if not scan["peak_indexes"]:
|
|
centre = np.mean(x)
|
|
else:
|
|
centre = x[int(scan["peak_indexes"])]
|
|
x = bin_data(x, binning)
|
|
y = list(scan["Counts"])
|
|
y_err = list(np.sqrt(y)) if scan.get("sigma", None) is None else list(scan["sigma"])
|
|
combined = bin_data(create_uncertanities(y, y_err), binning)
|
|
y = [combined[i].n for i in range(len(combined))]
|
|
y_err = [combined[i].s for i in range(len(combined))]
|
|
|
|
if len(scan["peak_indexes"]) == 0:
|
|
# Case for no peak, gaussian in centre, sigma as 20% of range
|
|
print("No peak")
|
|
peak_index = find_nearest(x, np.mean(x))
|
|
guess[0] = centre if guess[0] is None else guess[0]
|
|
guess[1] = (x[-1] - x[0]) / 5 if guess[1] is None else guess[1]
|
|
guess[2] = 50 if guess[2] is None else guess[2]
|
|
guess[3] = 0 if guess[3] is None else guess[3]
|
|
guess[4] = np.mean(y) if guess[4] is None else guess[4]
|
|
constraints_min[2] = 0
|
|
|
|
elif len(scan["peak_indexes"]) == 1:
|
|
# case for one peak, takse into account users guesses
|
|
print("one peak")
|
|
peak_height = scan["peak_heights"]
|
|
guess[0] = centre if guess[0] is None else guess[0]
|
|
guess[1] = 0.1 if guess[1] is None else guess[1]
|
|
guess[2] = float(peak_height / 10) if guess[2] is None else float(guess[2])
|
|
guess[3] = 0 if guess[3] is None else guess[3]
|
|
guess[4] = np.median(x) if guess[4] is None else guess[4]
|
|
constraints_min[0] = np.min(x) if constraints_min[0] is None else constraints_min[0]
|
|
constraints_max[0] = np.max(x) if constraints_max[0] is None else constraints_max[0]
|
|
|
|
def gaussian(x, g_cen, g_width, g_amp):
|
|
"""1-d gaussian: gaussian(x, amp, cen, wid)"""
|
|
return (g_amp / (np.sqrt(2 * np.pi) * g_width)) * np.exp(
|
|
-((x - g_cen) ** 2) / (2 * g_width ** 2)
|
|
)
|
|
|
|
def background(x, slope, intercept):
|
|
"""background"""
|
|
return slope * (x - centre) + intercept
|
|
|
|
mod = Model(gaussian) + Model(background)
|
|
params = Parameters()
|
|
params.add_many(
|
|
("g_cen", guess[0], bool(vary[0]), np.min(x), np.max(x), None, None),
|
|
("g_width", guess[1], bool(vary[1]), constraints_min[1], constraints_max[1], None, None),
|
|
("g_amp", guess[2], bool(vary[2]), constraints_min[2], constraints_max[2], None, None),
|
|
("slope", guess[3], bool(vary[3]), constraints_min[3], constraints_max[3], None, None),
|
|
("intercept", guess[4], bool(vary[4]), constraints_min[4], constraints_max[4], None, None),
|
|
)
|
|
# the weighted fit
|
|
try:
|
|
result = mod.fit(
|
|
y, params, weights=[np.abs(1 / val) for val in y_err], x=x, calc_covar=True,
|
|
)
|
|
except ValueError:
|
|
return
|
|
|
|
if result.params["g_amp"].stderr is None:
|
|
result.params["g_amp"].stderr = result.params["g_amp"].value
|
|
elif result.params["g_amp"].stderr > result.params["g_amp"].value:
|
|
result.params["g_amp"].stderr = result.params["g_amp"].value
|
|
|
|
# u.ufloat to work with uncertanities
|
|
fit_area = u.ufloat(result.params["g_amp"].value, result.params["g_amp"].stderr)
|
|
comps = result.eval_components()
|
|
|
|
if len(scan["peak_indexes"]) == 0:
|
|
# for case of no peak, there is no reason to integrate, therefore fit and int are equal
|
|
int_area = fit_area
|
|
|
|
elif len(scan["peak_indexes"]) == 1:
|
|
gauss_3sigmamin = find_nearest(
|
|
x, result.params["g_cen"].value - 3 * result.params["g_width"].value
|
|
)
|
|
gauss_3sigmamax = find_nearest(
|
|
x, result.params["g_cen"].value + 3 * result.params["g_width"].value
|
|
)
|
|
numfit_min = gauss_3sigmamin if numfit_min is None else find_nearest(x, numfit_min)
|
|
numfit_max = gauss_3sigmamax if numfit_max is None else find_nearest(x, numfit_max)
|
|
|
|
it = -1
|
|
while abs(numfit_max - numfit_min) < 3:
|
|
# in the case the peak is very thin and numerical integration would be on zero omega
|
|
# difference, finds closes values
|
|
it = it + 1
|
|
numfit_min = find_nearest(
|
|
x,
|
|
result.params["g_cen"].value - 3 * (1 + it / 10) * result.params["g_width"].value,
|
|
)
|
|
numfit_max = find_nearest(
|
|
x,
|
|
result.params["g_cen"].value + 3 * (1 + it / 10) * result.params["g_width"].value,
|
|
)
|
|
|
|
if x[numfit_min] < np.min(x):
|
|
# makes sure that the values supplied by user lay in the omega range
|
|
# can be ommited for users who know what they're doing
|
|
numfit_min = gauss_3sigmamin
|
|
print("Minimal integration value outside of x range")
|
|
elif x[numfit_min] >= x[numfit_max]:
|
|
numfit_min = gauss_3sigmamin
|
|
print("Minimal integration value higher than maximal")
|
|
else:
|
|
pass
|
|
if x[numfit_max] > np.max(x):
|
|
numfit_max = gauss_3sigmamax
|
|
print("Maximal integration value outside of x range")
|
|
elif x[numfit_max] <= x[numfit_min]:
|
|
numfit_max = gauss_3sigmamax
|
|
print("Maximal integration value lower than minimal")
|
|
else:
|
|
pass
|
|
|
|
count_errors = create_uncertanities(y, y_err)
|
|
# create error vector for numerical integration propagation
|
|
num_int_area = simps(count_errors[numfit_min:numfit_max], x[numfit_min:numfit_max])
|
|
slope_err = u.ufloat(result.params["slope"].value, result.params["slope"].stderr)
|
|
# pulls the nominal and error values from fit (slope)
|
|
intercept_err = u.ufloat(
|
|
result.params["intercept"].value, result.params["intercept"].stderr
|
|
)
|
|
# pulls the nominal and error values from fit (intercept)
|
|
|
|
background_errors = np.array([])
|
|
for j in range(len(x[numfit_min:numfit_max])):
|
|
# creates nominal and error vector for numerical integration of background
|
|
bg = slope_err * (x[j] - centre) + intercept_err
|
|
background_errors = np.append(background_errors, bg)
|
|
|
|
num_int_background = simps(background_errors, x[numfit_min:numfit_max])
|
|
int_area = num_int_area - num_int_background
|
|
|
|
d = {}
|
|
for pars in result.params:
|
|
d[str(pars)] = (result.params[str(pars)].value, result.params[str(pars)].vary)
|
|
print(result.fit_report())
|
|
|
|
print((result.params["g_amp"].value - int_area.n) / result.params["g_amp"].value)
|
|
|
|
d["ratio"] = (result.params["g_amp"].value - int_area.n) / result.params["g_amp"].value
|
|
d["int_area"] = int_area
|
|
d["fit_area"] = u.ufloat(result.params["g_amp"].value, result.params["g_amp"].stderr)
|
|
d["full_report"] = result.fit_report()
|
|
d["result"] = result
|
|
d["comps"] = comps
|
|
d["numfit"] = [numfit_min, numfit_max]
|
|
d["x_fit"] = x
|
|
scan["fit"] = d
|