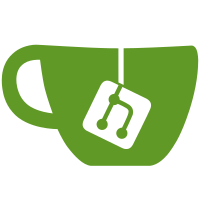
Add duplicate detection for spreadsheet data processing Implemented logic to detect and handle duplicate 'positioninpuck' entries within the same puck during spreadsheet processing. Updated backend to validate duplicates and provide detailed error messages. Enhanced frontend to visually highlight duplicate errors and allow better user feedback during cell editing. ```
357 lines
14 KiB
Python
357 lines
14 KiB
Python
import logging
|
|
import openpyxl
|
|
import re
|
|
from pydantic import ValidationError
|
|
from typing import List, Tuple
|
|
from io import BytesIO
|
|
from app.sample_models import SpreadsheetModel
|
|
|
|
logging.basicConfig(level=logging.DEBUG)
|
|
logger = logging.getLogger(__name__)
|
|
|
|
|
|
class SpreadsheetImportError(Exception):
|
|
pass
|
|
|
|
|
|
class SampleSpreadsheetImporter:
|
|
def __init__(self):
|
|
self.filename = None
|
|
self.model = None
|
|
|
|
def get_expected_type(self, column_name: str) -> type:
|
|
"""
|
|
Returns the expected data type for a given column name.
|
|
"""
|
|
# Define a mapping of column names to expected types
|
|
column_type_mapping = {
|
|
"dewarname": str,
|
|
"puckname": str,
|
|
"pucktype": str,
|
|
"crystalname": str,
|
|
"positioninpuck": int,
|
|
"priority": int,
|
|
"comments": str,
|
|
"proteinname": str,
|
|
"directory": str,
|
|
"oscillation": float,
|
|
"exposure": float,
|
|
"totalrange": int,
|
|
"transmission": int,
|
|
"targetresolution": float,
|
|
"aperture": str,
|
|
"datacollectiontype": str,
|
|
"processingpipeline": str,
|
|
"spacegroupnumber": int,
|
|
"cellparameters": str,
|
|
"rescutkey": str,
|
|
"rescutvalue": float,
|
|
"userresolution": float,
|
|
"pdbid": str,
|
|
"autoprocfull": bool,
|
|
"procfull": bool,
|
|
"adpenabled": bool,
|
|
"noano": bool,
|
|
"ffcscampaign": bool,
|
|
"trustedhigh": float,
|
|
"autoprocextraparams": str,
|
|
"chiphiangles": float,
|
|
"dose": float,
|
|
}
|
|
|
|
# Return type if column exists, else default to str
|
|
return column_type_mapping.get(column_name, str)
|
|
|
|
def _clean_value(self, value, expected_type=None, column_name=None):
|
|
"""
|
|
Cleans and validates the given value based on its expected type.
|
|
Tracks corrections and defaults applied separately.
|
|
"""
|
|
default_applied = False
|
|
# If the value is None or empty string
|
|
if value is None or (isinstance(value, str) and value.strip() == ""):
|
|
if column_name == "directory":
|
|
logger.warning("Directory value is empty. Assigning default value.")
|
|
default_applied = True
|
|
return "{sgPuck}/{sgPosition}", default_applied
|
|
elif column_name == "priority":
|
|
logger.warning("Priority value is empty. Assigning default value.")
|
|
default_applied = True
|
|
return 1, default_applied
|
|
return None, default_applied
|
|
|
|
# Clean up the value
|
|
cleaned_value = str(value).strip()
|
|
|
|
# Handle `type` casting logic
|
|
if expected_type == str:
|
|
if column_name == "comments":
|
|
return " ".join(cleaned_value.split()), default_applied
|
|
if " " in cleaned_value:
|
|
cleaned_value = cleaned_value.replace(" ", "_")
|
|
|
|
elif expected_type in [int, float]:
|
|
try:
|
|
cleaned_value = re.sub(r"[^\d.]", "", cleaned_value)
|
|
cleaned_value = expected_type(cleaned_value)
|
|
except (ValueError, TypeError) as e:
|
|
logger.error(
|
|
f"Failed to cast value '{value}' to {expected_type}. Error: {e}"
|
|
)
|
|
raise ValueError(
|
|
f"Invalid value: '{value}'. Expected type: {expected_type}."
|
|
)
|
|
|
|
# Avoid marking `None -> None` as a correction
|
|
if cleaned_value == value:
|
|
default_applied = (
|
|
False # Ensure default_applied stays False for unchanged `value`.
|
|
)
|
|
|
|
if not isinstance(cleaned_value, (str, int, float)):
|
|
raise TypeError(f"Unexpected type for cleaned value: {type(cleaned_value)}")
|
|
return cleaned_value, default_applied
|
|
|
|
def import_spreadsheet(self, file):
|
|
return self.import_spreadsheet_with_errors(file)
|
|
|
|
def import_spreadsheet_with_errors(
|
|
self, file
|
|
) -> Tuple[List[SpreadsheetModel], List[dict], List[dict], List[str]]:
|
|
self.model = []
|
|
self.filename = file.filename
|
|
logger.info(f"Importing spreadsheet from .xlsx file: {self.filename}")
|
|
|
|
contents = file.file.read()
|
|
file.file.seek(0) # Reset file pointer to the beginning
|
|
|
|
if not contents:
|
|
logger.error("The uploaded file is empty.")
|
|
raise SpreadsheetImportError("The uploaded file is empty.")
|
|
|
|
try:
|
|
workbook = openpyxl.load_workbook(BytesIO(contents))
|
|
logger.debug("Workbook loaded successfully")
|
|
if "Samples" not in workbook.sheetnames:
|
|
logger.error("The file is missing 'Samples' worksheet.")
|
|
raise SpreadsheetImportError("The file is missing 'Samples' worksheet.")
|
|
sheet = workbook["Samples"]
|
|
except Exception as e:
|
|
logger.error(f"Failed to read the file: {str(e)}")
|
|
raise SpreadsheetImportError(f"Failed to read the file: {str(e)}")
|
|
|
|
# Unpack four values from the process_spreadsheet method
|
|
model, errors, raw_data, headers = self.process_spreadsheet(sheet)
|
|
|
|
# Now, return the values correctly
|
|
return model, errors, raw_data, headers
|
|
|
|
def process_spreadsheet(
|
|
self, sheet
|
|
) -> Tuple[List[SpreadsheetModel], List[dict], List[dict], List[str]]:
|
|
model = []
|
|
errors = []
|
|
raw_data = []
|
|
|
|
rows = list(sheet.iter_rows(min_row=4, values_only=True))
|
|
logger.debug(f"Starting to process {len(rows)} rows from the sheet")
|
|
|
|
if not rows:
|
|
logger.error("The 'Samples' worksheet is empty.")
|
|
raise SpreadsheetImportError("The 'Samples' worksheet is empty.")
|
|
|
|
expected_columns = 32 # Number of columns expected based on the model
|
|
|
|
# Add the headers (the first row in the spreadsheet or map them explicitly)
|
|
headers = [
|
|
"dewarname",
|
|
"puckname",
|
|
"pucktype",
|
|
"crystalname",
|
|
"positioninpuck",
|
|
"priority",
|
|
"comments",
|
|
"directory",
|
|
"proteinname",
|
|
"oscillation",
|
|
"aperture",
|
|
"exposure",
|
|
"totalrange",
|
|
"transmission",
|
|
"dose",
|
|
"targetresolution",
|
|
"datacollectiontype",
|
|
"processingpipeline",
|
|
"spacegroupnumber",
|
|
"cellparameters",
|
|
"rescutkey",
|
|
"rescutvalue",
|
|
"userresolution",
|
|
"pdbid",
|
|
"autoprocfull",
|
|
"procfull",
|
|
"adpenabled",
|
|
"noano",
|
|
"ffcscampaign",
|
|
"trustedhigh",
|
|
"autoprocextraparams",
|
|
"chiphiangles",
|
|
]
|
|
|
|
duplicate_check = {}
|
|
|
|
for index, row in enumerate(rows):
|
|
if not any(row):
|
|
logger.debug(f"Skipping empty row at index {index}")
|
|
continue
|
|
|
|
# Ensure row has the expected number of columns
|
|
if len(row) < expected_columns:
|
|
row = list(row) + [None] * (expected_columns - len(row))
|
|
|
|
# Reset flags for the current row
|
|
self.default_set = False
|
|
corrected = False
|
|
defaulted_columns = []
|
|
corrected_columns = []
|
|
record = {}
|
|
|
|
for col_idx, column_name in enumerate(headers):
|
|
original_value = row[col_idx] if col_idx < len(row) else None
|
|
expected_type = self.get_expected_type(column_name)
|
|
|
|
try:
|
|
# Call `_clean_value` to clean the value and extract
|
|
# cleaning-related indicators
|
|
cleaned_value, default_applied = self._clean_value(
|
|
original_value, expected_type, column_name
|
|
)
|
|
|
|
# Check if the cleaned value is meaningfully different from the
|
|
# original value
|
|
is_corrected = cleaned_value != original_value
|
|
|
|
# Append column to corrected columns only if the value was corrected
|
|
if is_corrected:
|
|
corrected = True
|
|
corrected_columns.append(column_name)
|
|
|
|
# Track default columns separately if a default was applied
|
|
if default_applied:
|
|
corrected = True
|
|
defaulted_columns.append(column_name)
|
|
|
|
# Update the record with cleaned value
|
|
record[column_name] = cleaned_value
|
|
except (ValueError, TypeError) as e:
|
|
logger.error(
|
|
f"Validation error for row {index + 4},"
|
|
f" column '{column_name}': {str(e)}"
|
|
)
|
|
errors.append(
|
|
{
|
|
"row": index + 4,
|
|
"column": column_name,
|
|
"value": original_value,
|
|
"message": str(e),
|
|
}
|
|
)
|
|
|
|
# Validate duplicate 'positioninpuck' within the same puck
|
|
dewarname = record.get("dewarname")
|
|
puckname = record.get("puckname")
|
|
positioninpuck = record.get("positioninpuck")
|
|
|
|
if (
|
|
dewarname and puckname and positioninpuck is not None
|
|
): # Only check if all required fields exist
|
|
duplicate_key = f"{dewarname}-{puckname}"
|
|
if duplicate_key not in duplicate_check:
|
|
duplicate_check[duplicate_key] = set()
|
|
|
|
if positioninpuck in duplicate_check[duplicate_key]:
|
|
# Add error for duplicate position in the same puck
|
|
logger.warning(
|
|
f"Duplicate position '{positioninpuck}' found in puck"
|
|
f" '{puckname}' (dewar: '{dewarname}')"
|
|
)
|
|
errors.append(
|
|
{
|
|
"row": index + 4, # Adjust row number for 1-based indexing
|
|
"column": "positioninpuck", # The problematic column
|
|
"value": positioninpuck, # The value causing the issue
|
|
"message": f"Duplicate position '{positioninpuck}'"
|
|
f" found in puck '{puckname}' of dewar '{dewarname}'.",
|
|
}
|
|
)
|
|
|
|
else:
|
|
duplicate_check[duplicate_key].add(positioninpuck)
|
|
|
|
# Build metadata for the row
|
|
raw_data.append(
|
|
{
|
|
"row_num": index + 4,
|
|
"data": list(row), # Original data
|
|
"default_set": bool(
|
|
defaulted_columns
|
|
), # True if any defaults were applied
|
|
"corrected": corrected, # True if any value was corrected
|
|
"corrected_columns": corrected_columns,
|
|
"defaulted_columns": defaulted_columns,
|
|
}
|
|
)
|
|
|
|
# Nested processing for data_collection_parameters
|
|
record["data_collection_parameters"] = {
|
|
"directory": record.get("directory", ""),
|
|
"oscillation": record.get("oscillation", 0.0),
|
|
"aperture": record.get("aperture", None),
|
|
"exposure": record.get("exposure", 0.0),
|
|
"totalrange": record.get("totalrange", 0),
|
|
"transmission": record.get("transmission", 0),
|
|
"dose": record.get("dose", None),
|
|
"targetresolution": record.get("targetresolution", 0.0),
|
|
"datacollectiontype": record.get("datacollectiontype", None),
|
|
"processingpipeline": record.get("processingpipeline", None),
|
|
"spacegroupnumber": record.get("spacegroupnumber", None),
|
|
"cellparameters": record.get("cellparameters", None),
|
|
"rescutkey": record.get("rescutkey", None),
|
|
"rescutvalue": record.get("rescutvalue", 0.0),
|
|
"userresolution": record.get("userresolution", 0.0),
|
|
"pdbid": record.get("pdbid", ""),
|
|
"autoprocfull": record.get("autoprocfull", False),
|
|
"procfull": record.get("procfull", False),
|
|
"adpenabled": record.get("adpenabled", False),
|
|
"noano": record.get("noano", False),
|
|
"ffcscampaign": record.get("ffcscampaign", False),
|
|
"trustedhigh": record.get("trustedhigh", 0.0),
|
|
"autoprocextraparams": record.get("autoprocextraparams", None),
|
|
"chiphiangles": record.get("chiphiangles", 0.0),
|
|
}
|
|
|
|
try:
|
|
# Validate the record
|
|
validated_record = SpreadsheetModel(**record)
|
|
model.append(validated_record)
|
|
except ValidationError as e:
|
|
logger.error(f"Validation error in row {index + 4}: {e}")
|
|
for error in e.errors():
|
|
field_path = error["loc"]
|
|
msg = error["msg"]
|
|
column_name = headers[field_path[0]]
|
|
|
|
error_info = {
|
|
"row": index + 4,
|
|
"column": column_name,
|
|
"value": row[col_idx],
|
|
"message": msg,
|
|
}
|
|
errors.append(error_info)
|
|
|
|
logger.info(
|
|
f"Finished processing {len(model)} records with {len(errors)} errors"
|
|
)
|
|
|
|
return self.model, errors, raw_data, headers # Include headers in the response
|