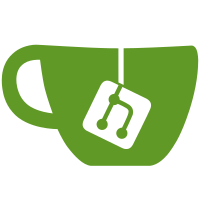
Implemented a toggleable spreadsheet UI component for sample data, added fields such as priority and comments, and improved backend validation. Default values for "directory" are now assigned when missing, with feedback highlighted in green on the front end.
257 lines
10 KiB
Python
257 lines
10 KiB
Python
import logging
|
|
import openpyxl
|
|
from pydantic import ValidationError
|
|
from typing import List, Tuple
|
|
from io import BytesIO
|
|
from app.sample_models import SpreadsheetModel
|
|
|
|
logging.basicConfig(level=logging.DEBUG)
|
|
logger = logging.getLogger(__name__)
|
|
|
|
|
|
class SpreadsheetImportError(Exception):
|
|
pass
|
|
|
|
|
|
class SampleSpreadsheetImporter:
|
|
def __init__(self):
|
|
self.filename = None
|
|
self.model = None
|
|
|
|
def _clean_value(self, value, expected_type=None):
|
|
"""Clean value by converting it to the expected type and handle edge cases."""
|
|
if value is None:
|
|
return None
|
|
if expected_type == str:
|
|
# Ensure value is converted to string and stripped of whitespace
|
|
return str(value).strip()
|
|
if expected_type in [float, int]:
|
|
try:
|
|
return expected_type(value)
|
|
except (ValueError, TypeError):
|
|
# If conversion fails, return None
|
|
return None
|
|
if isinstance(value, str):
|
|
try:
|
|
# Handle numeric strings
|
|
if "." in value:
|
|
return float(value)
|
|
else:
|
|
return int(value)
|
|
except ValueError:
|
|
pass
|
|
# In case of failure, return the stripped string
|
|
return value.strip()
|
|
# If no expected type or value type match, return the original value
|
|
return value
|
|
|
|
def import_spreadsheet(self, file):
|
|
return self.import_spreadsheet_with_errors(file)
|
|
|
|
def import_spreadsheet_with_errors(
|
|
self, file
|
|
) -> Tuple[List[SpreadsheetModel], List[dict], List[dict], List[str]]:
|
|
self.model = []
|
|
self.filename = file.filename
|
|
logger.info(f"Importing spreadsheet from .xlsx file: {self.filename}")
|
|
|
|
contents = file.file.read()
|
|
file.file.seek(0) # Reset file pointer to the beginning
|
|
|
|
if not contents:
|
|
logger.error("The uploaded file is empty.")
|
|
raise SpreadsheetImportError("The uploaded file is empty.")
|
|
|
|
try:
|
|
workbook = openpyxl.load_workbook(BytesIO(contents))
|
|
logger.debug("Workbook loaded successfully")
|
|
if "Samples" not in workbook.sheetnames:
|
|
logger.error("The file is missing 'Samples' worksheet.")
|
|
raise SpreadsheetImportError("The file is missing 'Samples' worksheet.")
|
|
sheet = workbook["Samples"]
|
|
except Exception as e:
|
|
logger.error(f"Failed to read the file: {str(e)}")
|
|
raise SpreadsheetImportError(f"Failed to read the file: {str(e)}")
|
|
|
|
# Unpack four values from the process_spreadsheet method
|
|
model, errors, raw_data, headers = self.process_spreadsheet(sheet)
|
|
|
|
# Now, return the values correctly
|
|
return model, errors, raw_data, headers
|
|
|
|
def process_spreadsheet(
|
|
self, sheet
|
|
) -> Tuple[List[SpreadsheetModel], List[dict], List[dict], List[str]]:
|
|
model = []
|
|
errors = []
|
|
raw_data = []
|
|
headers = []
|
|
|
|
# Skip the first 3 rows
|
|
rows = list(sheet.iter_rows(min_row=4, values_only=True))
|
|
logger.debug(f"Starting to process {len(rows)} rows from the sheet")
|
|
|
|
if not rows:
|
|
logger.error("The 'Samples' worksheet is empty.")
|
|
raise SpreadsheetImportError("The 'Samples' worksheet is empty.")
|
|
|
|
expected_columns = 32 # Number of columns expected based on the model
|
|
|
|
# Add the headers (the first row in the spreadsheet or map them explicitly)
|
|
headers = [
|
|
"dewarname",
|
|
"puckname",
|
|
"pucktype",
|
|
"crystalname",
|
|
"positioninpuck",
|
|
"priority",
|
|
"comments",
|
|
"directory",
|
|
"proteinname",
|
|
"oscillation",
|
|
"aperture",
|
|
"exposure",
|
|
"totalrange",
|
|
"transmission",
|
|
"dose",
|
|
"targetresolution",
|
|
"datacollectiontype",
|
|
"processingpipeline",
|
|
"spacegroupnumber",
|
|
"cellparameters",
|
|
"rescutkey",
|
|
"rescutvalue",
|
|
"userresolution",
|
|
"pdbid",
|
|
"autoprocfull",
|
|
"procfull",
|
|
"adpenabled",
|
|
"noano",
|
|
"ffcscampaign",
|
|
"trustedhigh",
|
|
"autoprocextraparams",
|
|
"chiphiangles",
|
|
]
|
|
|
|
for index, row in enumerate(rows):
|
|
if not any(row):
|
|
logger.debug(f"Skipping empty row at index {index}")
|
|
continue
|
|
|
|
# Record raw data for later use
|
|
raw_data.append({"row_num": index + 4, "data": row})
|
|
|
|
# Pad the row to ensure it has the expected number of columns
|
|
if len(row) < expected_columns:
|
|
row = list(row) + [None] * (expected_columns - len(row))
|
|
|
|
# Prepare the record with the cleaned values
|
|
record = {
|
|
"dewarname": self._clean_value(row[0], str),
|
|
"puckname": self._clean_value(row[1], str),
|
|
"pucktype": self._clean_value(row[2], str),
|
|
"crystalname": self._clean_value(row[3], str),
|
|
"positioninpuck": self._clean_value(row[4], int),
|
|
"priority": self._clean_value(row[5], int),
|
|
"comments": self._clean_value(row[6], str),
|
|
"directory": self._clean_value(row[7], str),
|
|
"proteinname": self._clean_value(row[8], str),
|
|
"oscillation": self._clean_value(row[9], float),
|
|
"aperture": self._clean_value(row[10], str),
|
|
"exposure": self._clean_value(row[11], float),
|
|
"totalrange": self._clean_value(row[12], float),
|
|
"transmission": self._clean_value(row[13], int),
|
|
"dose": self._clean_value(row[14], float),
|
|
"targetresolution": self._clean_value(row[15], float),
|
|
"datacollectiontype": self._clean_value(row[16], str),
|
|
"processingpipeline": self._clean_value(row[17], str),
|
|
"spacegroupnumber": self._clean_value(row[18], int),
|
|
"cellparameters": self._clean_value(row[19], str),
|
|
"rescutkey": self._clean_value(row[20], str),
|
|
"rescutvalue": self._clean_value(row[21], str),
|
|
"userresolution": self._clean_value(row[22], str),
|
|
"pdbid": self._clean_value(row[23], str),
|
|
"autoprocfull": self._clean_value(row[24], str),
|
|
"procfull": self._clean_value(row[25], str),
|
|
"adpenabled": self._clean_value(row[26], str),
|
|
"noano": self._clean_value(row[27], str),
|
|
"ffcscampaign": self._clean_value(row[28], str),
|
|
"trustedhigh": self._clean_value(row[29], str),
|
|
"autoprocextraparams": self._clean_value(row[30], str),
|
|
"chiphiangles": self._clean_value(row[31], str),
|
|
}
|
|
|
|
try:
|
|
validated_record = SpreadsheetModel(**record)
|
|
# Update the raw data with assigned default values
|
|
if (
|
|
validated_record.directory == "{sgPuck}/{sgPosition}"
|
|
and row[7] is None
|
|
):
|
|
row_list = list(row)
|
|
row_list[
|
|
7
|
|
] = validated_record.directory # Set the field to the default value
|
|
raw_data[-1]["data"] = row_list
|
|
raw_data[-1][
|
|
"default_set"
|
|
] = True # Mark this row as having a default value assigned
|
|
|
|
model.append(validated_record)
|
|
logger.debug(f"Row {index + 4} processed and validated successfully")
|
|
except ValidationError as e:
|
|
logger.error(f"Validation error in row {index + 4}: {e}")
|
|
for error in e.errors():
|
|
field = error["loc"][0]
|
|
msg = error["msg"]
|
|
# Map field name (which is the key in `record`) to its index in the
|
|
# row
|
|
field_to_col = {
|
|
"dewarname": 0,
|
|
"puckname": 1,
|
|
"pucktype": 2,
|
|
"crystalname": 3,
|
|
"positioninpuck": 4,
|
|
"priority": 5,
|
|
"comments": 6,
|
|
"directory": 7,
|
|
"proteinname": 8,
|
|
"oscillation": 9,
|
|
"aperture": 10,
|
|
"exposure": 11,
|
|
"totalrange": 12,
|
|
"transmission": 13,
|
|
"dose": 14,
|
|
"targetresolution": 15,
|
|
"datacollectiontype": 16,
|
|
"processingpipeline": 17,
|
|
"spacegroupnumber": 18,
|
|
"cellparameters": 19,
|
|
"rescutkey": 20,
|
|
"rescutvalue": 21,
|
|
"userresolution": 22,
|
|
"pdbid": 23,
|
|
"autoprocfull": 24,
|
|
"procfull": 25,
|
|
"adpenabled": 26,
|
|
"noano": 27,
|
|
"ffcscampaign": 28,
|
|
"trustedhigh": 29,
|
|
"autoprocextraparams": 30,
|
|
"chiphiangles": 31,
|
|
}
|
|
column_index = field_to_col[field]
|
|
error_info = {
|
|
"row": index + 4,
|
|
"cell": column_index,
|
|
"value": row[column_index], # Value that caused the error
|
|
"message": msg,
|
|
}
|
|
errors.append(error_info)
|
|
|
|
self.model = model
|
|
logger.info(
|
|
f"Finished processing {len(model)} records with {len(errors)} errors"
|
|
)
|
|
return self.model, errors, raw_data, headers # Include headers in the response
|