mirror of
https://github.com/slsdetectorgroup/aare.git
synced 2025-04-21 14:20:02 +02:00
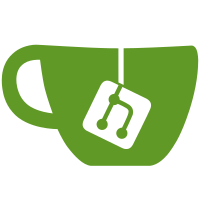
- added stand alone fitting using: https://jugit.fz-juelich.de/mlz/lmfit.git - fit_gaus, fit_pol1 with and without errors - multi threaded fitting --------- Co-authored-by: JulianHeymes <julian.heymes@psi.ch>
80 lines
2.6 KiB
Python
80 lines
2.6 KiB
Python
import matplotlib.pyplot as plt
|
||
import numpy as np
|
||
from aare import fit_gaus, fit_pol1
|
||
from aare import gaus, pol1
|
||
|
||
textpm = f"±" #
|
||
textmu = f"μ" #
|
||
textsigma = f"σ" #
|
||
|
||
|
||
|
||
# ================================= Gauss fit =================================
|
||
# Parameters
|
||
mu = np.random.uniform(1, 100) # Mean of Gaussian
|
||
sigma = np.random.uniform(4, 20) # Standard deviation
|
||
num_points = 10000 # Number of points for smooth distribution
|
||
noise_sigma = 100
|
||
|
||
# Generate Gaussian distribution
|
||
data = np.random.normal(mu, sigma, num_points)
|
||
|
||
# Generate errors for each point
|
||
errors = np.abs(np.random.normal(0, sigma, num_points)) # Errors with mean 0, std 0.5
|
||
|
||
# Create subplot
|
||
fig0, ax0 = plt.subplots(1, 1, num=0, figsize=(12, 8))
|
||
|
||
x = np.histogram(data, bins=30)[1][:-1] + 0.05
|
||
y = np.histogram(data, bins=30)[0]
|
||
yerr = errors[:30]
|
||
|
||
|
||
# Add the errors as error bars in the step plot
|
||
ax0.errorbar(x, y, yerr=yerr, fmt=". ", capsize=5)
|
||
ax0.grid()
|
||
|
||
par, err = fit_gaus(x, y, yerr)
|
||
print(par, err)
|
||
|
||
x = np.linspace(x[0], x[-1], 1000)
|
||
ax0.plot(x, gaus(x, par), marker="")
|
||
ax0.set(xlabel="x", ylabel="Counts", title=f"A0 = {par[0]:0.2f}{textpm}{err[0]:0.2f}\n"
|
||
f"{textmu} = {par[1]:0.2f}{textpm}{err[1]:0.2f}\n"
|
||
f"{textsigma} = {par[2]:0.2f}{textpm}{err[2]:0.2f}\n"
|
||
f"(init: {textmu}: {mu:0.2f}, {textsigma}: {sigma:0.2f})")
|
||
fig0.tight_layout()
|
||
|
||
|
||
|
||
# ================================= pol1 fit =================================
|
||
# Parameters
|
||
n_points = 40
|
||
|
||
# Generate random slope and intercept (origin)
|
||
slope = np.random.uniform(-10, 10) # Random slope between 0.5 and 2.0
|
||
intercept = np.random.uniform(-10, 10) # Random intercept between -10 and 10
|
||
|
||
# Generate random x values
|
||
x_values = np.random.uniform(-10, 10, n_points)
|
||
|
||
# Calculate y values based on the linear function y = mx + b + error
|
||
errors = np.abs(np.random.normal(0, np.random.uniform(1, 5), n_points))
|
||
var_points = np.random.normal(0, np.random.uniform(0.1, 2), n_points)
|
||
y_values = slope * x_values + intercept + var_points
|
||
|
||
fig1, ax1 = plt.subplots(1, 1, num=1, figsize=(12, 8))
|
||
ax1.errorbar(x_values, y_values, yerr=errors, fmt=". ", capsize=5)
|
||
par, err = fit_pol1(x_values, y_values, errors)
|
||
|
||
|
||
x = np.linspace(np.min(x_values), np.max(x_values), 1000)
|
||
ax1.plot(x, pol1(x, par), marker="")
|
||
ax1.set(xlabel="x", ylabel="y", title=f"a = {par[0]:0.2f}{textpm}{err[0]:0.2f}\n"
|
||
f"b = {par[1]:0.2f}{textpm}{err[1]:0.2f}\n"
|
||
f"(init: {slope:0.2f}, {intercept:0.2f})")
|
||
fig1.tight_layout()
|
||
|
||
plt.show()
|
||
|